Can Large Language Models (LLM) Assist Neurologists in Analyzing Stroke Victims?
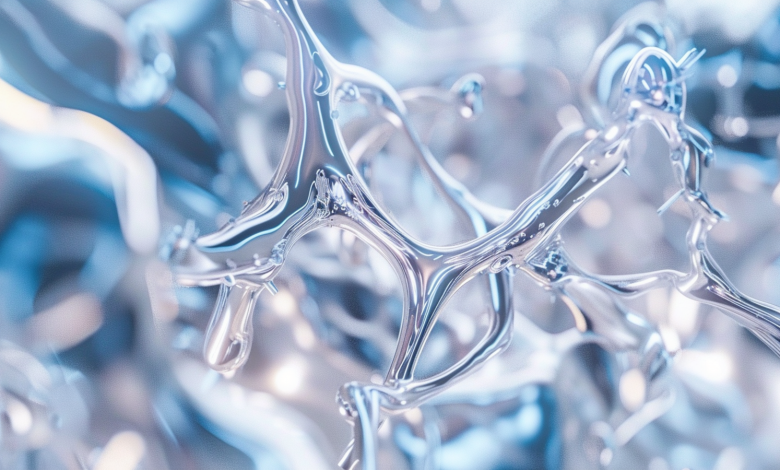
Artificial intelligence (AI) is one of the biggest technological advances of this decade. With the launch and popularity of ChatGPT, AI has gone mainstream, finding its application across various sectors, including healthcare. In this realm, neurologists can leverage AI to pinpoint the location of strokes within the brain, as revealed by a recent study.
İçindekiler
Strokes are a leading cause of death among Americans; every 40 seconds, someone in the US experiences a stroke, and every 3 minutes and 14 seconds, someone dies from one. Beyond mortality, strokes can also cause long-term disabilities. Therefore, identifying the stroke’s location within the brain can help predict its effects in the long run, which can vary widely, from impairing an individual’s mobility to affecting language and speech abilities. Additionally, AI can help determine a person’s prognosis and determine the best treatment.
Typically, brain scans help find the location of lesions, which are areas of tissue damage from strokes. However, not everyone can have access to neurologists for brain scans.
To address this gap, “we wanted to determine whether GPT-4 could accurately locate brain lesions after stroke based on a person’s health history and a neurologic exam,” said study author Jung-Hyun Lee, MD, a member of the American Academy of Neurology and the State University of New York, Downstate Health Sciences University in Brooklyn.
Other participants of the study include Eunhee Choi, MD, Department of Internal Medicine, Lincoln Medical Center, NY; Robert McDougal, MD, Department of Biostatistics at Yale School of Public Health and Program in Computational Biology and Bioinformatics at Wu-Tsai Institute; and William W. Lytton, MD, Department of Neurology at State University of New York Downstate Health Sciences University and Department of Neurology at Kings County Hospital.
Published in the American Academy of Neurology’s online journal, Neurology® Clinical Practice, the study showcased the capability of AI to process text from neurologic examinations and health histories to identify the lesion location in the brain.
For this, the team used the large language model (LLM) GPT-4. This generative pre-trained transformer is trained on huge amounts of data and has shown “remarkable capabilities” in generating text responses to text-based queries.
This endeavor is not the first of its kind, as the functionality of LLMs has previously been explored in answering free-form posed by physicians. However, previous software developed for lesion localization has been rather limited in scope. The aim of this study is to evaluate the capability of GPT -4 in the localization of acute stroke lesions based on clinical presentation, thereby exhibiting the potential role AI can play as a clinical tool in neurology for the future.
Huge Potential
In this study, researchers compiled the health histories and neurologic physical exams of 46 published cases of those who had a stroke. This textual data was then fed into GPT-4. Utilizing Zero-Shot Chain-of-Thought and Text Classification prompting techniques, the model was tasked with answering three specific questions about each patient, repeated three times: whether a participant had single or multiple lesions, on which side of the brain lesions were located, and in which region of the brain the lesions were found.
Upon comparing the GPT -4 results with the brain scans of each participant, researchers found that the results were pretty satisfactory, except for lesions in the cerebellum and spinal cord.
GPT-4 demonstrated a high degree of accuracy in identifying the location of the brain lesions for the majority of cases, achieving a specificity of 87% (percentage of negatives correctly identified) and 74% sensitivity (percentage of actual positives correctly identified). The model also achieved a specificity of 94% and a sensitivity of 85% while determining the most affected brain regions.
The study further found that the model consistently identified the number of brain lesions in 76% of participants. the location of lesions in 83%, and the specific brain regions involved in 87% of cases. However, when evaluating the model’s responses to all three questions across three attempts for each participant, its consistency drastically declined, with GPT-4 only able to provide accurate answers for 41% of participants.
This indicates that LLMs, such as generative pre-trained transformers (GPT), are “not yet ready for use in the clinic.” Nonetheless, they show promise in identifying the location of lesions post-stroke and could reduce healthcare disparities, thanks to their ability to function across different languages.
The study also highlights the growing importance of neurologic expertise for stroke intervention, especially with a surge in telemedicine usage. Furthermore, the model can assist in timely decisions to assess the need for more imaging studies or even neurologist consultation.
According to Lee:
“Their potential for use is encouraging, especially due to the great need for improved health care in underserved areas across multiple countries where access to neurologic care is limited.”
Notably, GPT-4’s accuracy depends on the quality of data it is fed, and detailed information about medical history and neuro exams may not always be available for everyone who has a stroke.
The study emphasized that the researchers have been working with an LLM without medical training or fine-tuning. Additionally, it was noted that the reports of atypical cases often lacked adequately detailed health histories and neurologic examinations. Thus, inaccuracies arose from insufficient information in the published cases, as well as failures of logic or gaps in the underlying knowledge base.
So, there is a need for further research in terms of logistical, legal, patient safety and privacy, ensuring accuracy and consistency, and integration with hospital workflows for LLM’s viable utilization in clinical settings.
Click here to learn why 2023 was a breakout year for artificial intelligence.
Growing Importance & Usage of LLMs in Healthcare
The study shows great results, showcasing yet another example of LLMs’ expanding role in healthcare—a field that has been gaining much attention. After all, they are changing the sector in so many ways. Their use is gradually moving from exploration stages to practical use, now impacting clinical research and patient care. Their capacity to process voluminous data, offer real-time insights, and produce text that is of human quality and with certain professional knowledge is revolutionizing the sector.
Trained on large amounts of complex data, including text, images, and videos obtained from the internet, these models find versatile applications across various areas, including in medicine.
It first started gaining traction when Microsoft-backed OpenAI released its first LLM, GPT-1, in 2018. Its widespread adoption was driven by its public accessibility and convenient usability. These features were the result of incorporating the model reinforcement learning from human feedback (RLHF), which helped it provide more credible and human-like output than its previous counterparts.
Since the launch of the LLM-based chatbot application ChatGPT, several tech companies, such as Meta (Llama), Google (BARD), Amazon, and Salesforce-backed Anthropic (Claude), have released their LLMs. Meanwhile, OpenAI’s latest version, GPT-4, has been showing enhanced capabilities, such as surpassing the passing score of the US Medical Licensing Exam (USMLE).
With that, ChatGPT has shown its ability for medical reasons, so by fine-tuning LLMs and providing them with additional training, we can use LLMs to improve patient care. Human communication is a key part of it, GPT’s human-like capability has allowed it to be pretty effective in patient satisfaction and enabling optimal clinical outcomes.
While processing huge amounts of data can be daunting for humans, LLMs can easily do this in a fraction of the time. They streamline this process by organizing the data, allowing us to make good use of it. Not just past data, either; LLMs continuously analyze new, incoming data such as vital signs and patient symptoms that can greatly assist healthcare professionals in making real-time and fast decisions.
By analyzing vast datasets from scientific literature, clinical trials, treatment guidelines, and medical records, LLMs are also speeding up medical research to help identify new treatments and develop effective therapies. These models can also help make correlations and detect patterns that can advance our understanding of diseases and accelerate medical research.
Moreover, these models provide valuable insights that help healthcare professionals make clinical decisions, such as accurate diagnoses and personalized treatment planning, leading to improved patient care. Not just professionals but LLMs also empower patients by providing them with understandable and accessible information to better understand their conditions and enable them to participate in their healthcare journeys.
Click here to learn what makes AI a jack of all trades.
Driving the Innovation in Healthcare
Given the vast benefits and potential, the healthcare generative AI market achieved a valuation of over $1 billion in 2022 and is expected to grow to $22 bln by 2032, as per the Generative AI Tracker.
When it comes to AI’s usage in the medical device sector, cardiology has been leading this trend, with 57% of AI-enabled medical devices approved by the FDA in 2022 to be under cardiology, according to GlobalData. This trend is expected to continue in the future, with the spending on AI by medical device companies set to grow at a 20.6% CAGR between 2019 and 2024.
In the future, we could even see the integration of AI with 3D bioprinting to enable the creation of fully functional organs tailored to every patient’s specific needs.
This potential has many mainstream organizations launching specialized AI-powered solutions for the healthcare industry. For instance, Microsoft provides a specialized solution called the Nuance Dragon Ambient eXperience (DAX). It captures clinician-patient conversations, translates them into notes, and integrates them into EHRs.
Google has already unveiled its MedLM, a healthcare-focused LLM available via the Vertex AI platform. The company has since been training its health AI models on its latest research to apply AI to the healthcare industry. The model has been used by partner organizations to build solutions such as streamlining nurse handoffs and supporting clinicians’ documentation.
Just last month, Google added novel abilities to its model and launched MedLM for Chest X-rays to change the way radiology works. The idea is to have generative AI support healthcare organizations by assisting with the categorization of chest X-rays for a number of use cases.
But this is not all. Google Research is working with Fitbit to build a large language model for personal health that can power wellness features in the Fitbit mobile app. This model is being worked on to offer individualized coaching to everyone based on their personal health and fitness goals.
Amazon Web Services (AWS) also offers HealthScribe, which analyzes consultation conversations to create summarized clinical notes for segments like assessment, history of present illness, and treatment plan. Organizations can then integrate these notes into clinical applications to recap the patient visits more efficiently.
Earlier this year, medical technology company Siemens Healthineers joined forces with the Indian Institute of Science (IISc) to launch the Siemens Healthineers-Computational Data Sciences (CDS) Collaborative Laboratory for AI in Precision Medicine. The Laboratory’s focus is on developing open-source AI tools to automate the precise segmentation of pathological findings in neuroimaging data. This will help with the more accurate diagnosis of diseases related to neurology and the analysis of their clinical effect.
This is just the beginning of the growing AI trend in the medical sector. The role of artificial intelligence is expected to be much bigger. Currently being used to generate real-time, patient-specific 3D models in surgeries, AI is set to evolve further to offer interactive healthcare experiences to transform patient education and engagement. Even in genome editing, AI is expected to move beyond just analyzing complex genetic data to provide more accurate gene-disease association predictions for highly tailored gene editing.
AI-powered immersive virtual reality therapy sessions for mental health treatment is another way the technology can be utilized in the sector. Then, there are nanobots that can leverage AI to identify and address a wide range of medical conditions at their early stages.
However, the application of LLMs in healthcare isn’t without its concern. There are ethical concerns in terms of privacy, transparency, and security considerations that researchers, healthcare professionals, and medical organizations must be mindful of. These models can also be used to distribute misinformation due to a lack of accountability, something that should also be taken into account. Not to mention, there is an inherent limitation of reproducing existing biases.
Governments around the world are working on tackling the issues posed by AI. Europe has already created a legal framework for AI, while the UK Department of Health and Social Care is planning to improve the transparency of data used in the development of medical devices using AI.
Click here to learn about the growing synergy of AI & neurology.
Conclusion
LLMs are already seeing growing usage in the medical industry, and they are expected to further grow in terms of adoption and impact. However, it is important that these models are used responsibly and the underlying data is of supreme quality without any inherent biases and misinformation to provide more accurate results and better patient outcomes.
Click here to learn all about investing in artificial intelligence.